Leveraging growth in the golden quarter: What is a good ROAS, and why does iROAS™ matter more?
In the ever-evolving world of online advertising, ROAS (return on advertising spend) is the compass guiding retailers to success – but relying solely on this metric has limitations.
The all-important golden quarter is around the corner, and retailers are gearing up for their most lucrative sales period – or is it? In 2022 alone, businesses funnelled a staggering £446 million into advertising during this time, but how much of that advertising spend actually generated return for retailers? And how much of that return was profitable?
Whether you’re measuring ROAS, or even profitable return on ad spend (POAS, PROAS), all of these metrics have one thing in common – they’re generally based on revenue, not true return. Only when you take into account all the associated costs – beyond just advertising – for each product do you really know your true return on ad spend.
In this blog, we look at ROAS in the world of Google Shopping; what a good ROAS truly is, why ROAS isn’t enough, and why iROAS™ is the formula every retailer should be using this golden quarter.
What is a good ROAS on Google Shopping?
In Google Shopping, ROAS is calculated by total sales revenue resulting from ad clicks divided by the total amount spent on Google Shopping ads.
An average ROAS on Google Shopping can be anywhere from 2:1 to 10:1, and a good ROAS is anything of 4:1 or higher.
However, even if you’re hitting this ROAS, it may not be an accurate reflection of how well you’re performing. A good ROAS varies hugely depending on what your goals are – you might be looking to shift some end-of-season stock, so revenue will be your main priority, or you may be looking to increase your margins in which case profit is your main objective. YOU know this, Google does not…
In short, a good ROAS looks different for every SKU depending on the goal – so having to use the same average ROAS target across your entire Google inventory is, as you well know, a dangerous decision to make.
Here’s why.
Firstly, ROAS begins at zero so if a campaign or a product is generating negative return on ad spend – you can’t see it.
Relying only on positive returns is dangerous as you may overlook any performance issues that occur below the breakeven point (0 ROAS) and conceal areas where retail strategies may be struggling. In store you’re constantly assessing the performance of every product, whereas on Google, ROAS targets do not allow you to see these issues.
Secondly, to get a more comprehensive evaluation of overall return, you need to consider additional costs involved in selling each product – from raw materials and manufacturing all the way through to import fees and taxes.
This is why it’s important to consider each individual product, not just product line or campaign when setting ROAS targets; because each product has its own break-even point.
Why ROAS is no longer good enough and iROAS matters more
Only when you look at these factors can you calculate your true return on ad spend.
ROAS traditionally focuses on revenue generated, rather than return. PROAS (profitable return on ad spend, aka POAS) takes this further by taking into account all the associated costs that go with selling a product – but it’s an incredibly complex, laborious and largely manual task.
iROAS™ is a complete solution for calculating true return on ad spend in this era of AI and automation in retail – and it requires zero manual work.
iROAS™ noun intelligent return on ad spend
Upp.’s intelligent return on ad spend (iROAS™) revolves around harnessing data around the individual SKUs that make up a retailer’s inventory. Upp.AI groups every SKU into optimised Google Shopping campaigns based on:
- Sales velocity – how likely the product will sell based on market conditions
- ROAS – the desired return on ad spend a retailer is looking to achieve for this product
These campaigns are optimised by Upp.AI using custom budgets, and the platform uses real-world data to ensure your products gain maximum visibility, based on your budget and goals (revenue vs profit vs ROAS).
Though businesses have access to this data, it’s impossible to connect, analyse and action this data at scale and with multiple SKUs in real time – all the time. Using machine learning to use iROAS™ means retailers can establish the exact break-even point for every individual product, which would otherwise be impossible to achieve at scale.
This allows for far more accurate decision-making across not only marketing and advertising but also promotions and discounts, alleviating any room for error or overbidding.
Upp.AI ensures that you are selling more stock, whilst minimising wasted spend – making it the most important tool in your arsenal when preparing for the Black Friday period.
Supercharge your ROAS with iROAS
As retailers brace themselves for the high stakes and intense discounting of Black Friday, Cyber Monday, and the holiday season, the importance of knowing that you’re achieving a good ROAS for each of your products is critical.
Let Upp.AI handle the impossible task of constant management and optimisation of your core Google Shopping campaigns 24/7 while you focus on your overall strategy. iROAS™ will give you the competitive advantage you’ve been looking for.
Gone are the days of ‘set and forget’ campaigns. Wouldn’t it be great to be able to trust that when you increase your ad budgets on Google Shopping, you know you’ll get a good return?
Upp.AI does exactly that. With iROAS™, you are finally able to control your Google Shopping campaigns so that you achieve the results that are fully aligned with your goals – like fashion retailer Roman who achieved a 181% increase in revenue.
It’s time to level up your Google Shopping campaigns, use technology where it can really help you and don’t let your competitors beat you this golden quarter. The only way to make Google Shopping work effectively for you is to embrace the change and make the move to AI and automation.
Get yourself ready for the biggest quarter of the year, and take the lead, just like these early adopters of AI and machine learning technology.
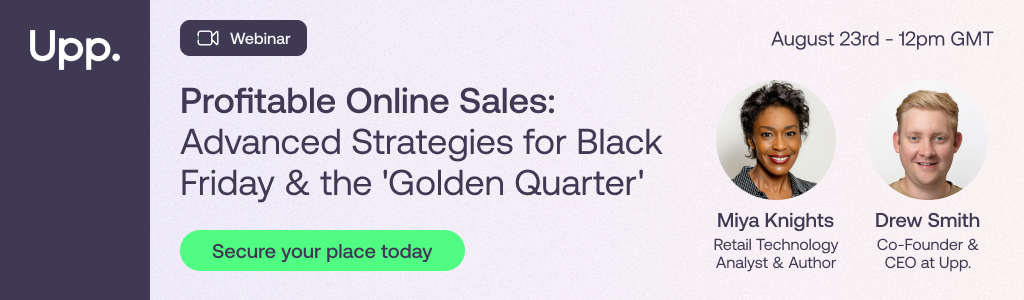